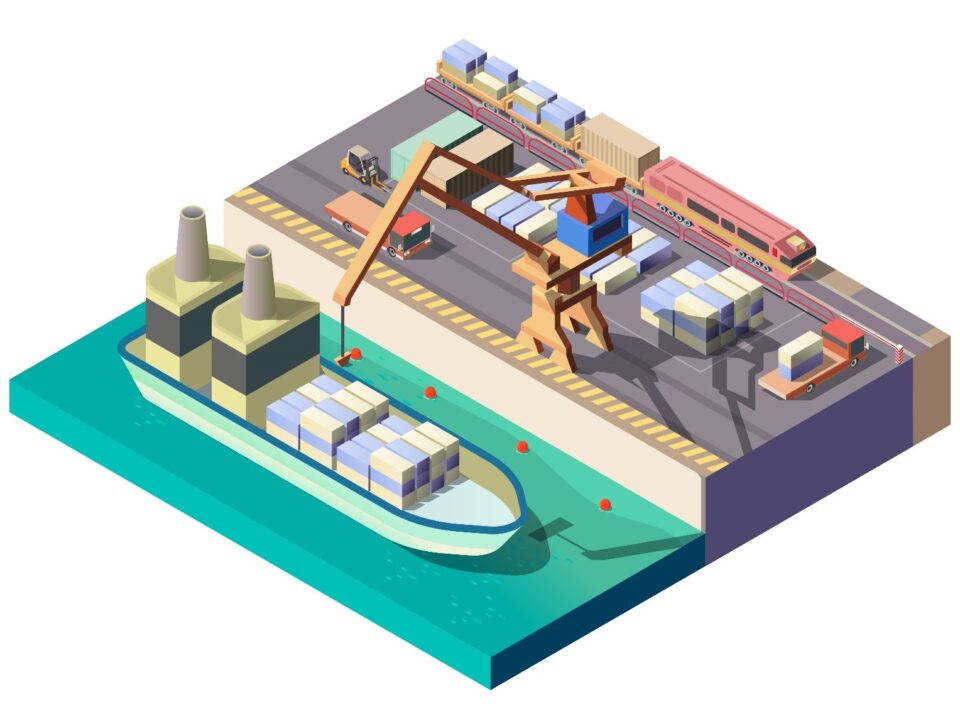
Climate crisis, energy transition, new mobility, digitization, e-commerce boom, demographic growth, material transition, national and supranational strategic lines… are challenges and opportunities typical of this day to which the ports of the world are looking for an answer.
Ports’ strategic responses to these challenges and opportunities include digitization and innovation, to such an extent that they are becoming hubs of innovation and data-driven organizations.
Where there is data and decisions must be made, advanced analytics is unavoidable.
The arrival of 5G and the Internet of Things (IoT) offers us unprecedented connectivity and sensorization. The rapid digitization of transport and logistics networks, and the interconnection of different systems and agents, have allowed information to flow from end to end in the port environment: from the port authority to the terminal operator, to the shipping company, and to the container depot, almost in real-time.
This flood of data, encouraged by connectivity and technology, stimulates the application of Advanced Analytics (Machine Learning, Artificial Intelligence, Mathematical Optimization) to find mathematical solutions to problems of discrete events simulation, forecasting, allocation, optimization, and planning, throughout the entire value chain. This post will speak over some areas of use.
Terminal operation design
At some point, any complex system requires challenging decisions that do not allow prior experimentation and which consequences, once made, are costly to rectify. A simple example is where to locate a factory. It is not a decision that allows a trial and error approach. An incorrect location will put a burden on the income statement, either due to excessive costs of installation, operation, logistics, etc.
This kind of decision requires a careful prior analysis, which can be so complex due to the interdependencies between the different parts of the system that in practice it is impossible.
Advanced analytics offers the chance to simulate the system. Simulation consists of the construction of a digital replica (a model) of the studied system with all the required detail in each of its elements, including the operational processes, in such a way that it allows observing the expected behavior of the system against different configurations.
This model is called a Digital Twin. Unfortunately, this term is often used to refer to a digital replica of system elements in order to display its configuration and performance information. In this case, we are dealing with a completely descriptive tool (a digital mirror), without simulation capabilities.
To the ports, these decisions may concern their design (location of different elements) or their processes. A Digital Twin provides a test environment to evaluate and compare changes without investment in equipment or interference in daily operations:
- Study of the system capacity in the face of new demand scenarios: bottlenecks and idle capacity.
- Evaluation of design and operation alternatives
- Acquisition of new equipment
- Extension of service provision hours
- Changes in the way of operating:
- new container storage strategies
- loading / unloading windows, etc.
The Digital Twin takes advantage of the historical data available for the use case under consideration: demand, operational processes, etc. In addition, in the IoT environment, the Digital Twin can be fed the information provided by the available sensors: location, pressure, temperature, vibration, etc.
Real-time operations management
Advanced analytics is a tool specially designed to offer solutions to complex problems, with a large number of factors and restrictions, such as the management of a port.
Disciplines such as Operational Research allow setting objectives such as maximizing the number of ships, trains and trucks served, increasing the productivity of the teams and reducing congestion, improving the visibility and traceability of the pass of containers, or minimizing the time of stay of these.
To do this, it combines public domain data such as meteorological information with specific data such as navigation routes and schedules and information on the infrastructures, equipment, and available spaces. With the information of the existing operating rules and thanks to the IoT, the location of each container and crane at any time, use cases are developed in some areas.
- Ship operations
- forecast of delays/early entrance in the arrival of ships
- assignment of ships to docks
- assignment of loading/unloading equipment to ships
- assigning tasks to teams considering the simultaneous arrival of trucks and trains
- etc.
- Truck operations
- loading / unloading zone assignment
- resource allocation for upload/download
- etc.
- Train operations
- need for manoeuvre
- assignment of unloading / loading path
- etc.
- Container yard
- traffic management
- assigning tasks to teams moving between zones
- etc.
The mix of these use cases offers extreme-to-end optimization of container transfer and storage in real-time.
Prescriptive maintenance
Prescriptive maintenance combines Machine Learning and Optimization to estimate the probability of equipment failure and improve the planning of maintenance tasks, making use of the incident history and information provided by the sensors located on the equipment.
In order to reduce maintenance costs and minimize the number of corrective maintenance, prescriptive maintenance is not limited to indicating a probability of equipment failure, but optimizes the monthly scheduling and annual planning of maintenance tasks, adapting them on the fly to changes in equipment conditions.
This use case shows the power of using predictive analytics and optimization together. These techniques not only show us the future but also propose the best mathematically calculated decision.