If you work in manufacturing or production processes, Federated Learning (FL) should be on your radar. In the era of Industry 4.0 and 5.0, where every process generates sensitive data, such as intellectual property and customer information, FL is revolutionizing the way we safeguard and leverage this critical resource.
This cutting-edge technology is growing fast. In 2023, the global FL market is projected to hit $130 million, with estimates soaring to $328 million by 2032. These figures underscore its disruptive potential for industries looking to scale securely and efficiently.
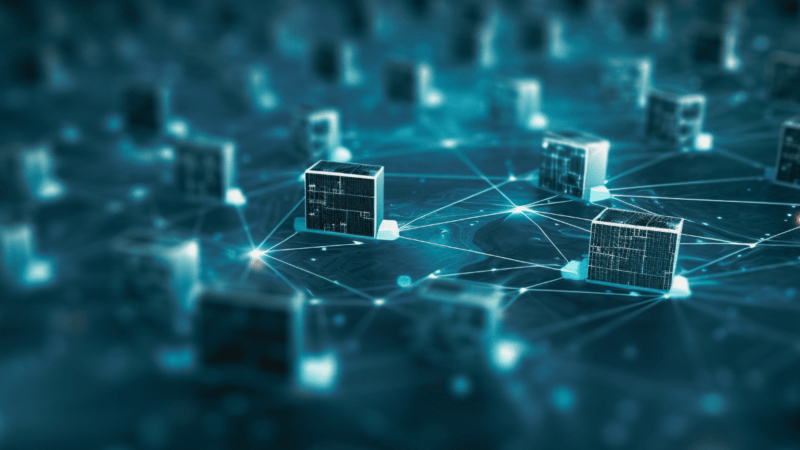
Why should FL matter to you and your business?
Federated Learning delivers strategic advantages that tackle core needs: privacy, security, and decision-making efficiency. By keeping data at its source, FL provides a collaborative framework for training AI models without exposing sensitive information. Below are some key benefits that FL can bring to your business:
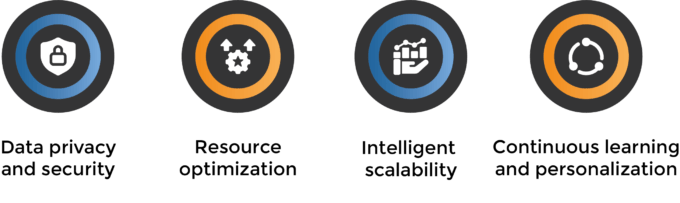
Data privacy and security: FL ensures that data never leaves its local device, meeting regulatory requirements and reducing the risk of sensitive information breaches.
Resource optimization: Instead of transferring raw data, only model updates are shared, speeding up processes and minimizing bandwidth usage.
Intelligent scalability: FL adapts seamlessly to different devices, factories, or regions without requiring centralized infrastructure, promoting growth and efficiency.
Continuous learning and personalization: Models are continuously updated based on local data, improving performance in specific environments while retaining the advantages of a global model.
How does FL work?
Here’s an overview of the FL process:
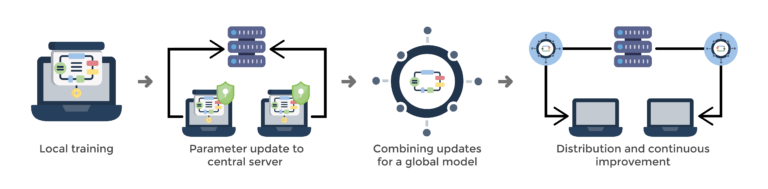
- Local training: Each device uses its own data to train a model independently, identifying unique patterns in its production environment without sharing sensitive information.
- Parameter updates to a central server: Only the model updates are sent, not raw data. This enables collaborative training while maintaining privacy.
- Combining updates for a global model: The central server aggregates updates from all devices to optimize a global model, which is then redistributed to improve accuracy at each location.
- Distribution and continuous improvement: The refined global model is sent back to devices, where it continues to learn and improve, enhancing operations while safeguarding data.
How is Federated Learning revolutionizing key Industries?
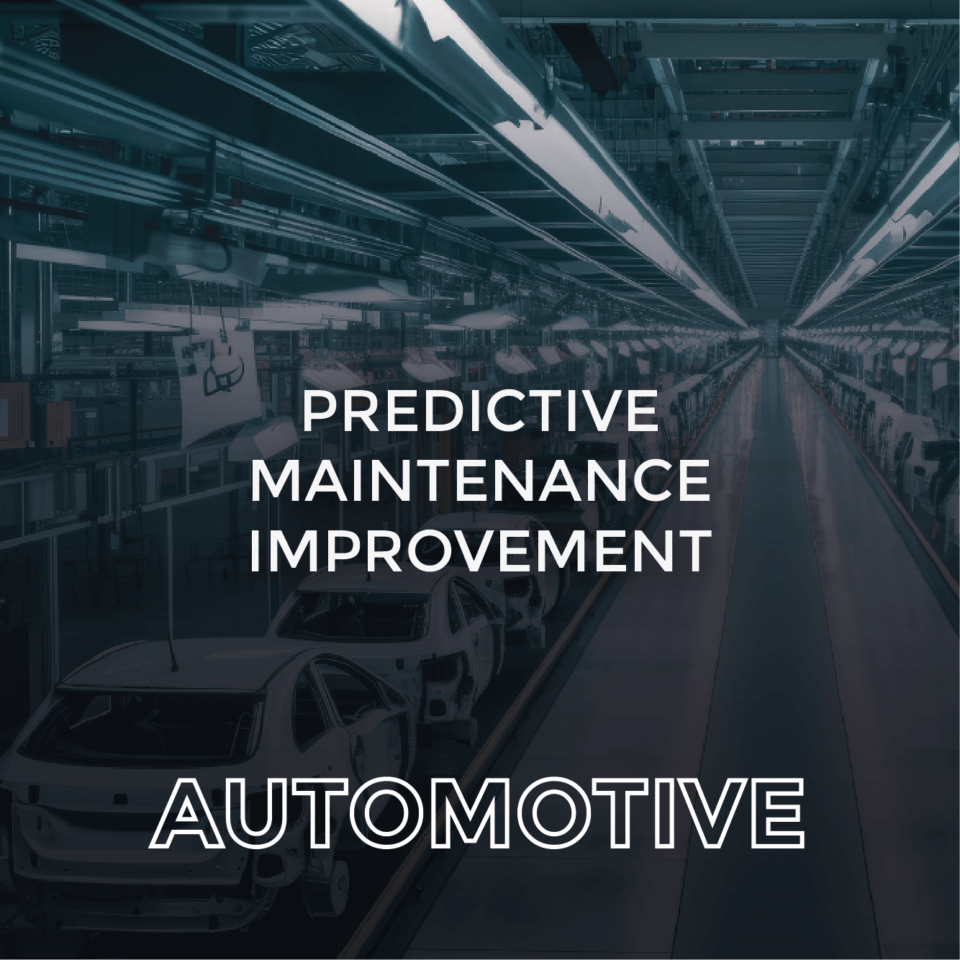
Automotive factories use FL to predict equipment failures without exposing sensitive data, enhancing the accuracy and efficiency of preventative maintenance.
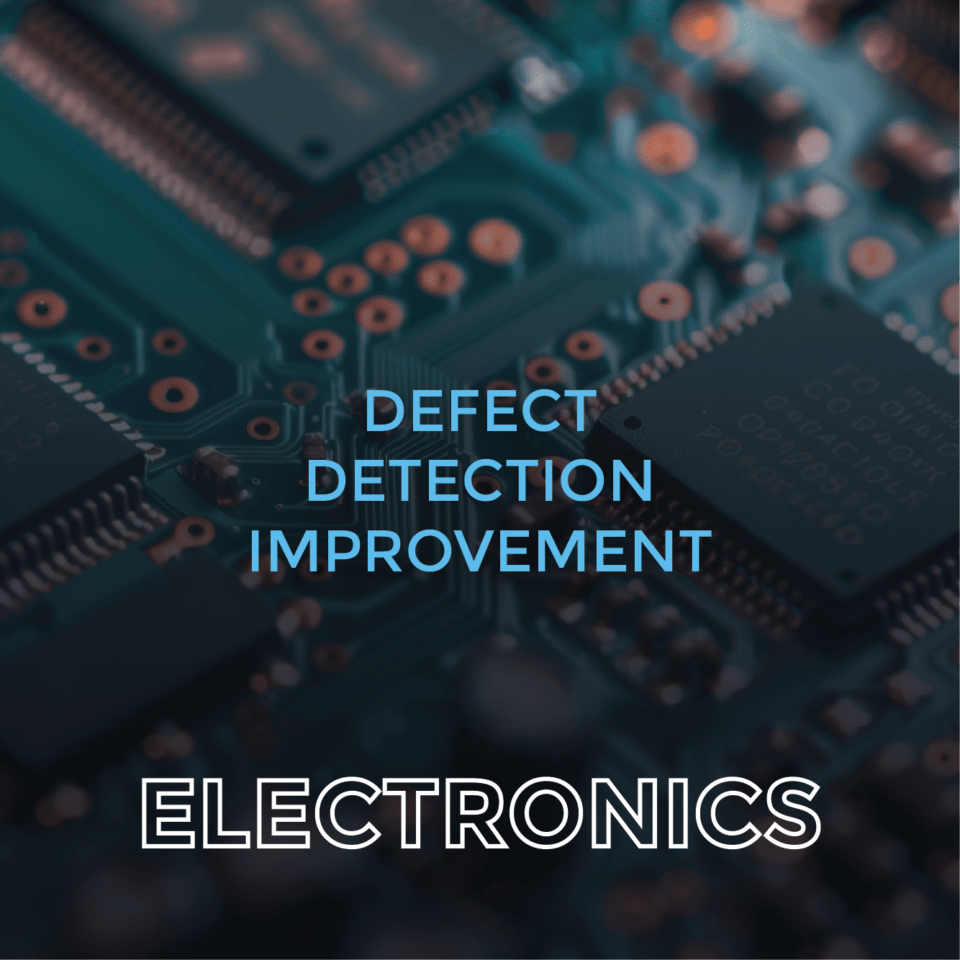
Each factory trains its model with local defect data, improving detection rates and minimizing waste.
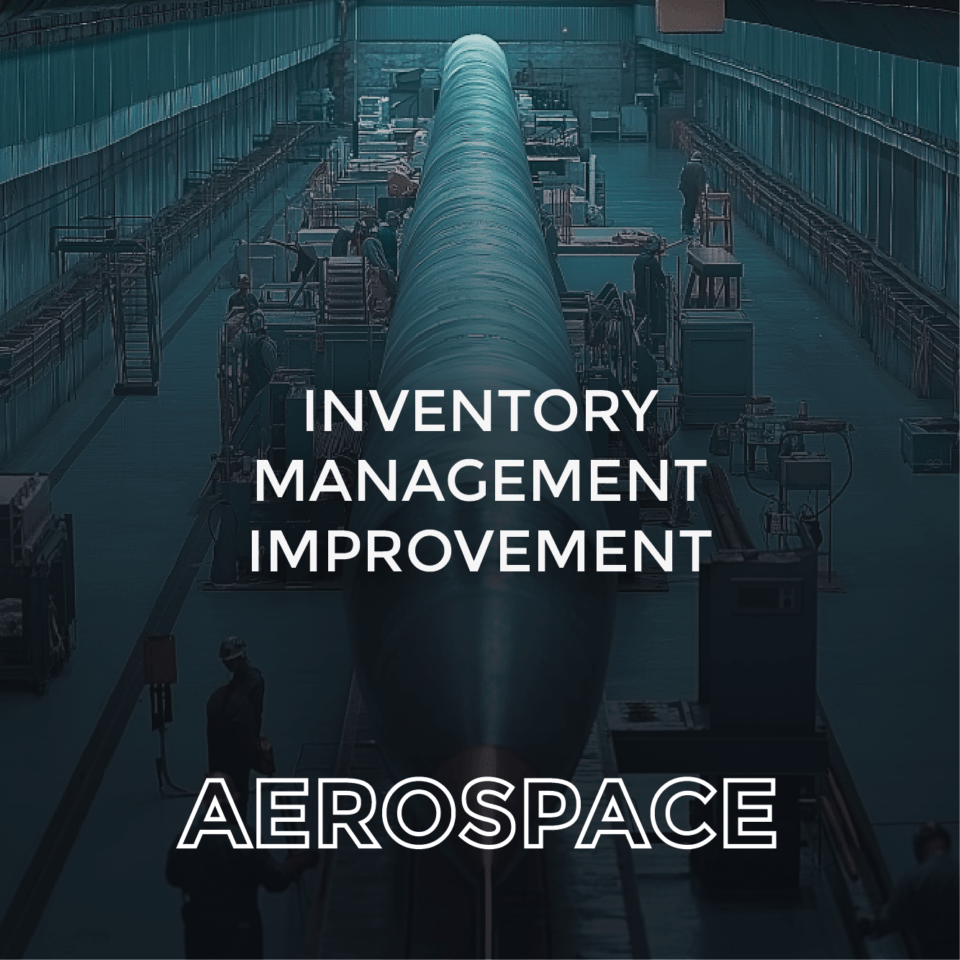
Suppliers and manufacturers in the aerospace supply chain collaboratively optimize inventories without sharing private data, reducing surplus stock and minimizing shortages.

Multiple textile plants leverage FL to optimize energy use, lowering operational costs and improving sustainability.
Ready to maximize your data and optimize your processes?
Boost your business with customized Opti AI solutions that easily integrate into your processes.
If you’re looking for a strategic partner to guide you through this technological transformation, consider working with experts who will support you every step of the way, from identifying challenges to implementing and monitoring AI solutions.
Schedule your free Smart Session today and transform your challenges into business opportunities.
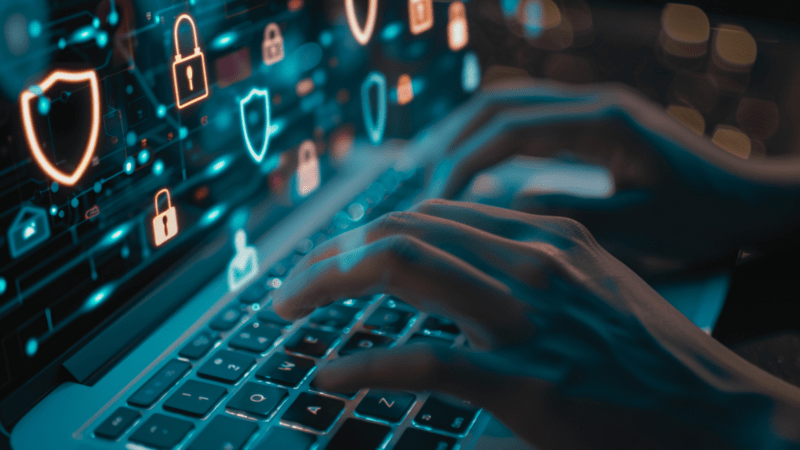
Adopting Federated Learning tools not only ensures the privacy of your data but also opens the door to new opportunities for innovation and efficiency. Discover how this approach can turn your challenges into competitive advantages.