
Although trading is usually connected with the stock market, in which Prescriptive Analytics is used extensively to optimize purchases and sales of securities such as stocks or bonds, it also exists in other markets, such as the energy market.
The trading departments of energy companies specialize in the purchase and sale of energy – a strategic activity – and although the fundamentals of these operations show many common characteristics, there are differences given by the sector, the function, the market and the mode of operation: Electricity trading is not the same as that of Oil & Gas, nor that of a trading company as that of a power generation company, nor is it the same in all the markets in which they operate, nor is it independent of corporate conditions, etc.
Improve trading decisions
These differences in the trading departments make even more interesting to us, Prescriptive Analytics professionals, the technical and mathematical challenge of applying our discipline to support purchase and sale decisions.
Energy traders make decisions every day regarding how much energy to buy or sell, at what prices, in which markets, whether it is spot, futures, options, etc. In addition, the decision environment is increasingly faster and different for each energy company. These are complex decisions that require complex tools and techniques with a very high degree of sophistication in order to offer the optimal solution.
As there are no off-the-shelf applications that cover all the casuistry and that are continuously updated for each organization, energy companies build their own trading platforms integrating third-party software with their own developments or those of external companies.
Optimisation with Prescriptive Analytics
Can energy buying and selling decisions really be improved through Prescriptive Analytics techniques? Definitely. Compared to Predictive Analytics, which can help predict energy demand, prices, etc., Prescriptive Analytics proposes the mathematically optimal (or near-optimal) decision out of a large number of possible decisions. This represents an improvement over decision-making based on human experience, which in most cases is not optimal.
It is very common to confuse Prescriptive Analytics with the application of if-then rules or decision trees to the results of Predictive Analytics (if X is predicted, Y is done). The use of decision trees in some energy trading environments can help to homogenize the decision criteria of traders, however it does not propose optimal decisions because the if-then rules do not explore the whole solution space.
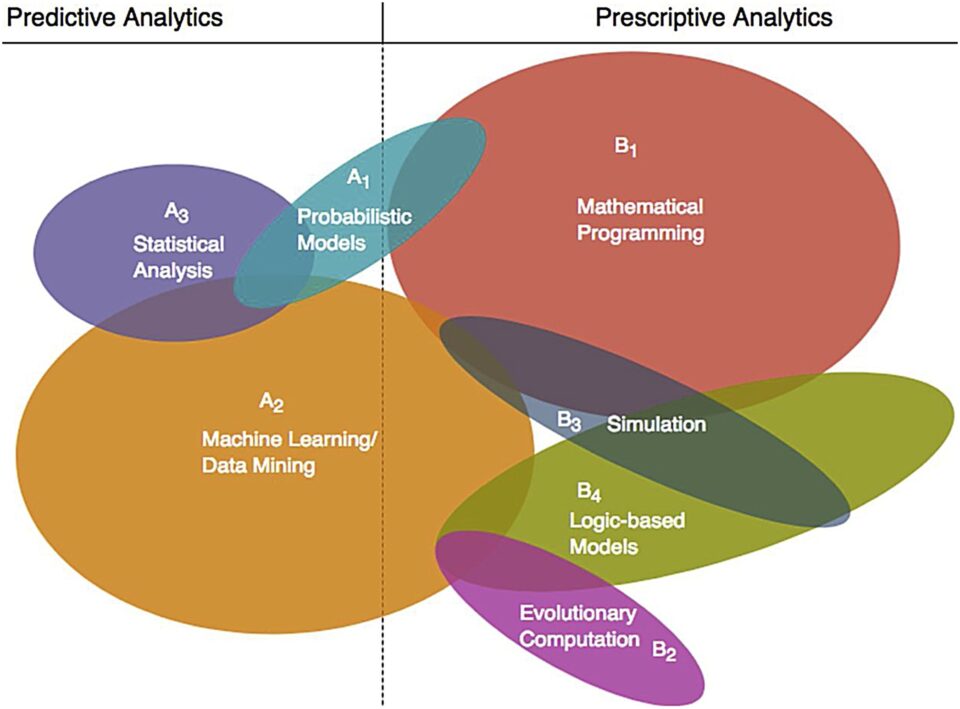
Predictive Analytics and Prescriptive Analytics.
Source: Lepenioti, Katerina, Alexandros Bousdekis, D. Apostolou and G. Mentzas. “Prescriptive analytics: Literature review and research challenges.” Int. J. Inf. Manag. 50 (2020): 57-70.
In contrast, Prescriptive Analytics finds the mathematically optimal decision using more elaborate techniques typical of Operations Research such as:
- Exact methods: optimal or close to optimal solutions.
- Decomposition based on exact methods (Benders, column generation, etc.)
- Stochastic linear programming.
- Heuristic decompositions, combination of simulation and heuristics (simheuristics).
- Constructive heuristics, local search, etc.
- Other metaheuristics.
- Simulated annealing.
- Ant Colony Optimisation
- Genetic algorithms
- Taboo searches
- Etc.
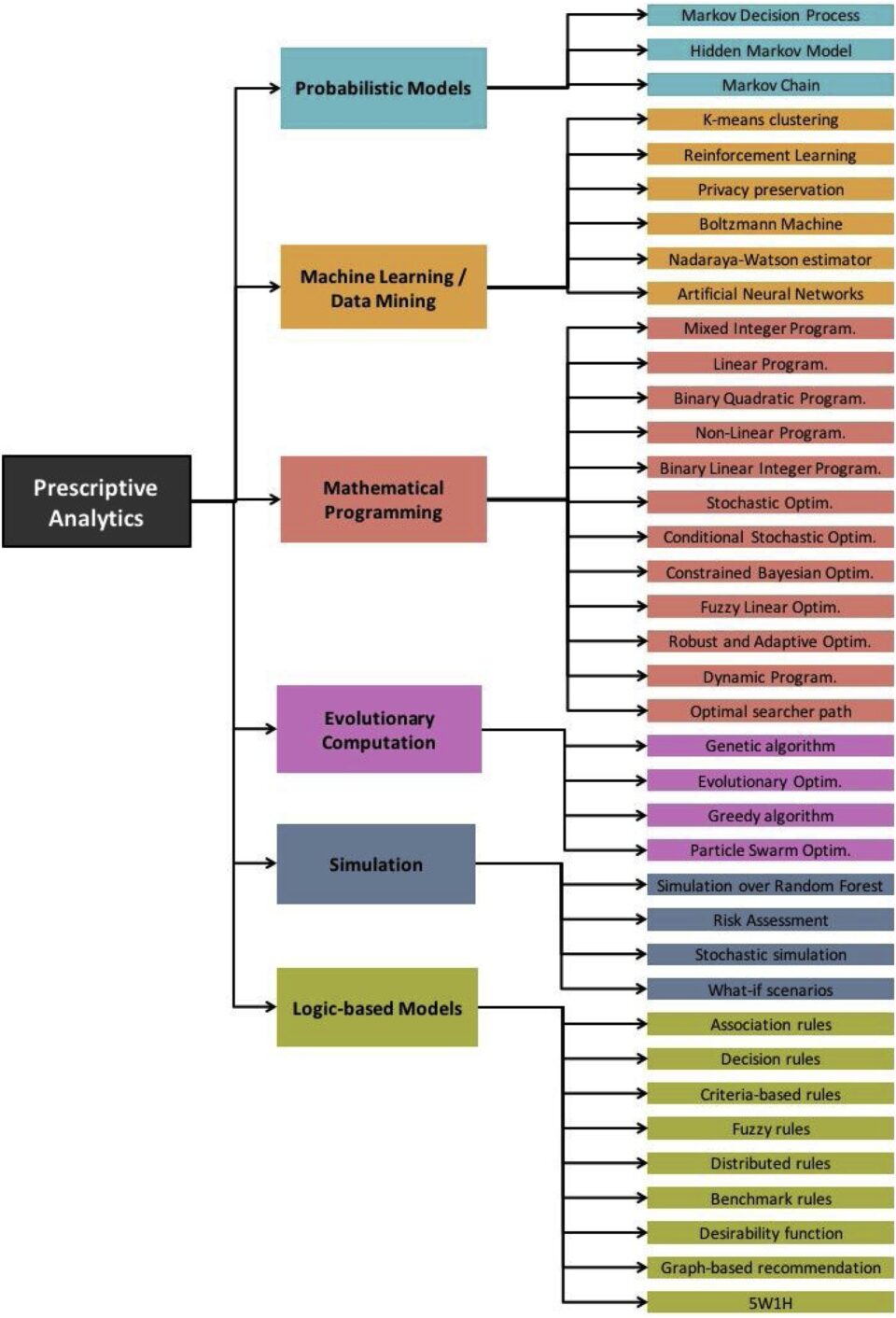
Prescriptive Analytics Techniques.
Source: Lepenioti, Katerina, Alexandros Bousdekis, D. Apostolou and G. Mentzas. “Prescriptive analytics: Literature review and research challenges.” Int. J. Inf. Manag. 50 (2020): 57-70.
When Predictive Analytics is not enough
In the field of trading, predictions of variables such as energy demand, generation / production, prices, etc., are not very accurate when the markets are very volatile or exceptional circumstances occur. They could even be totally wrong. We saw a paradigmatic example during the first weeks of confinement by COVID-19, when historical data were not good to predict the future and predictive models had to be turned off, which did not happen with Prescriptive Analytics models.
There are several techniques that are used to soften the variability of predictions within Predictive Analytics, however, with Prescriptive Analytics, this variability can be treated in a more efficient and controlled way by using, for example, stochastic linear programming, which works with probability distributions that represent said prediction variability.
Therefore, Prescriptive Analytics takes into account the variability of predictions to make mathematically optimal decisions even in the absence of a significant amount of data.
This makes Prescriptive Analytics a very useful tool to support energy trading decision making.
How to incorporate or promote the use of Prescriptive Analytics in trading departments
Trading departments have improved in recent years thanks to the incorporation of Data Science specialists in their teams, creating increasingly useful predictive models. Now is the time to go one step further, applying or reinforcing the use of Prescriptive Analytics techniques.
For this, you must have specialized support in Prescriptive Analytics / Operations Research, with proven experience in:
- The latest algorithms and techniques.
The open source or commercial tools that are most appropriate for each project.
- Effective and efficient mathematical formulation that allows obtaining solutions in the required time.
- Incorporate data from Predictive Analytics, and use Prescriptive Analytics to improve predictions and to soften their variability if necessary.
Last but not least, another key to the success of projects aiming to improve trading operations is in the creation of multidisciplinary working groups with:
The department’s energy trading specialists.
- In-house data scientists.
- Prescriptive Analytics specialists.
In-house vs outsourced.
Prescriptive Analytics is a demanding speciality that requires specific and continuous training and study. Not all Data Science groups have the capacity or the means to offer such competence development to their members.
In the stock market it is common to have very expensive quants or Quantitative Analysis teams on staff to support traders, given their high returns and incessant optimisation, but in other sectors the most common option is to outsource this competition.
Companies specialized in Prescriptive Analytics such as baobab soluciones are the optimal option to access the best talent and take advantage of their experience solving problems in a multitude of sectors.
If you need to improve your energy purchase and sale decisions, contact us at info@baobabsoluciones.es and we will be happy to help you.