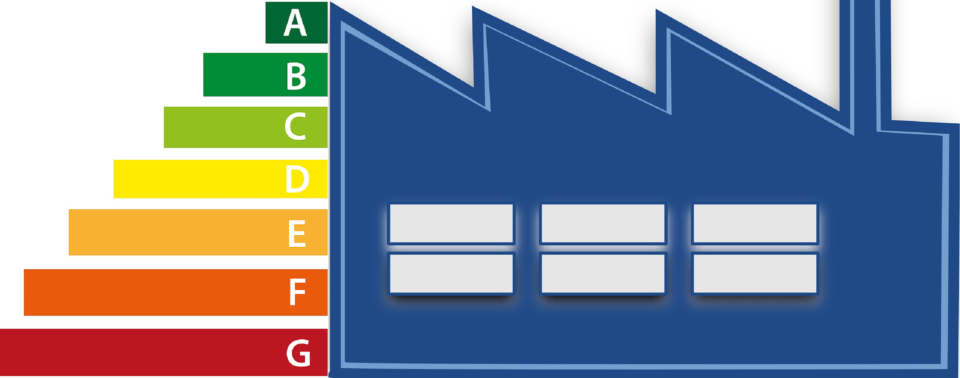
Efficiency is like magic. It allows you to achieve the same results with fewer resources and it is a profitability boost in business contexts. A 10% cost reduction in a 15%-margin business returns the same profit as a 56% increase in revenue. Who doesn’t want that?
One cost element where efficiency is especially welcome is the electricity bill because it adds environmental benefits to the purely financial savings. Mathematical optimisation, analytics and automation are of great help to reduce it.
A highly automated factory
This is a story about one of our customers. Its business was based in highly automated production plants, which worked 24×7. These plants used very little raw matter, a very thin staff, and electricity consumption was one of the top items in their cost base. They needed to increase their efficiency and competitiveness, but there was not much to reduce.
Enter baobab. We set out to help them reduce their electricity bill by understanding the inner workings of one of their production plants and translating it into mathematics.
How did advanced analytics help?
The main KPI of this activity is the energy cost per produced unit. Energy usage depends on the activity of the plant. Plants can operate differently at different states, each one being characterized by certain equipment running according to particular specifications. Every state is pegged to known production and energy consumption rates. Naturally, the higher the production rate, the higher the energy consumption.
This was not a trivial problem to solve. Energy contracts are quite complex and prices can vary significantly over time. Roughly, the power bill comprises two elements: a consumption cost with a fixed and a variable component, and a bonus for power usage in specific times of the day. The Spanish electricity market is arranged in such a manner that it incentivizes large consumers (such as production plants) to restrict power usage within certain periods by means of discounts.
Before baobab was involved, this task was carried out virtually by hand on an Excel spreadsheet, which took an exceeding amount of time to the Production Planner and despite being doable, it did not follow the changes in prices and regulations and could not adapt easily to changes in demand.
For that purpose, baobab experts developed a solution that assisted the Production Planner with the creation of the production plan with the lowest possible power cost. The tool was based on a Mixed Integer Linear Programming model for minimizing the energy consumption, subject to the following constraints:
• Customers’ demand must be met.
• Produced inventory must remain within the allowed levels.
• Only feasible transitions between states can be permitted.
• Meet minimum duration in a gear once started.
• The power bill must be computed according to contract.
• Changes between certain states imply an additional cost. Some of these changes required human intervention.
• The plant can be stopped any time. There is a cost for starting up the plant after a stop.
The model inputs included all regulations, energy prices and the production targets. It was inserted in an application that returned the hourly production plan. It was inserted in an application that returned the hourly production plan.
Results
The application yielded benefits on different levels:
• First and foremost, savings were in the mid six-figure range, while meeting all the production targets.
• Accurate planning, smoothly integrated in the plant control system.
• Reduced complexity and faster scheduling allowed the production managers to run new simulations and what-if analysis, thus anticipating previously unforeseen scenarios. e.g. Did it make sense to keep a person by night to execute certain changes or would the cost exceed the benefit?
• Last, it also proved as a live trial before taking advantage of scale and extending the solution to the other factories.
This project let machines do what they do best: quick calculations, while it allowed humans to take strategic roles and to think about the business goals, not the math. It consequently proved the value of mathematical optimization to unlock efficiency gains and reduce power usage in chains of production, while coping with demand and keeping the same level of quality.