Industry 4.0 is presented as the promised land of the 21st century in which the enormous information available and the interconnection between a multitude of elements allow for more effective and efficient operations: IoT and sensors, RFID tags, Real Time Location Services (RTLS), wearables… will collect and share their information with higher speed and lower latency through private 5G networks, displayed in real time on screens in control centres and AR glasses.
But the data is not enough, even if it is top quality and available in previously unimaginable amounts. This great data collection machine must serve some purpose: to guide the steps of the operators in a warehouse, to anticipate the failure of an element in the chain of production, or to prevent a stock-out in a warehouse.
Data is at the service of decision making
Data is at the service of decisions, be they operational, tactical or strategic. Strategic decisions usually allow time. We do not want to use just five minutes to decide on the location of a new plant (which will compromise our activity for many years). Besides, this is a very occasional type of decision.
However, when we must launch a production order, decide which is the next destination on our route or decide the movements of the AGVs in a logistics platform, five minutes is an unaffordable luxury.
In an operational context it is essential to make decisions quickly and data is not enough. We also need to process that information and know how our system operates to make a good decision. Furthermore, we need to make many good decisions repeatedly over time.
In operational terms it is no longer so important to achieve the optimum, as if it were the holy grail. Contexts change rapidly and “optima” cease to be so just as quickly. Therefore, looking for a monolithic plan does not make sense: if the context changes and the plan does not, the optimum we will get will no longer be valid. For example, the moment an incident occurs or an urgent order arrives or there is an unexpected traffic problem, the plan will no longer work and re-planning will be required.
That is why we are interested in algorithms that respond to changes as they occur, “agile” algorithms that offer sufficiently good solutions in a very short time and make use of the most recent information available in real time.
Until now, this type of task has been delegated to an expert, who needed to adjust swiftly to the changes in the environment. This expert is the one who stores all the past and present knowledge about the system in their brain: experience embodied.
However, dependence on this guru introduces a weakness not only for the most obvious reasons (when that person is missing, the system operates very poorly) but for other subtle yet important ones. For example, reviewing the way of operation may not possible if the expert cannot assess the impact of certain changes in the operation without advanced analytical tools.
The good news is that the groundwork is laid for prescriptive analytics, which is not a newcomer. It has a long tradition and new techniques or refinements of the existing ones do not cease to appear: from the first one during the 50s (with stars such as Danzig and his Linear Programming) through the appearance of bio-inspired algorithms (such as ant colonies, genetic algorithms) and recently, the combination of prescriptive analytics and machine learning.
Just in Time production scheduling, which reduces stock and inventory costs to a minimum, or energy consumption optimization in industrial environments are classic prescriptive analytics use cases.
Now there are two new ingredients: real-time data and computing power. With them and with prescriptive analytics it is now possible to offer at all times the best solution required by the systems. There are many experiences that show the savings and the impact offered by the transition to the 4.0 world.
Even the skeptical manager who is afraid of delegating on an algorithm, specially in a complex and uncertain environment, can learn of its advantages and “buy insurance” before this transition thanks to a digital twin. By means of simulation it is possible to build a digital replica, a model of your system, with all the necessary detail to analyze the impact of possible decisions before applying them in a safe and agile environment.
For example, if we are talking about a logistics platform, we can represent in detail the reception and unloading of pallets, the classification, the stock replenishment, etc.
Once the digital twin is available, it is possible to explore any scenarios, in particular, the introduction of all the IoT sensors and the related algorithms necessary for the exploitation of all the information they provide.
That is, in the previous example, we can evaluate the impact of switching from a manual picking process to a process using AGVs governed with advanced analytics. This analysis does not require advanced investment in sensors, AGV or the introduction of any type of change. These changes can be made in the digital model and include the algorithms that would operate the system once those changes are made. So, we can see the results of the change in the process before undertaking the investments.
This approach presents two great advantages. On the one hand, it allows us to study different alternatives before putting them into practice (with our digital model we can analyze as many alternatives as needed). On the other hand, it is possible to anticipate the ROI of the investment for each alternative without any risk and subsequently to bring the most convenient option to our system, with a high degree of certainty on the outcome.
The use of the digital model together with IoT and real-time algorithms is valid for a very broad set of contexts: production planning and control, delivery routes, preventive maintenance, supply management, parking traffic management … Let’s see some examples .
Smart stock management
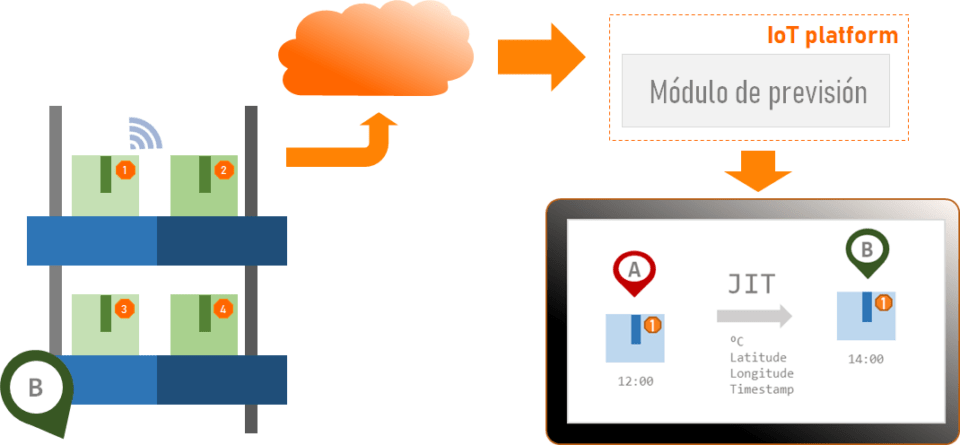
Smart or connected stock management enables Just in Time sourcing through 1) constant monitoring of supplies and stock, 2) demand forecast, which feeds an optimization algorithm that adjusts the supply orders to the expected demand, considering all the pertinent restrictions.
The advantage of monitoring is that in the event of an incident affecting supply (for example, cold chain break), detection is practically immediate and the best possible corrective action is launched as quickly as possible.
Delivery routes
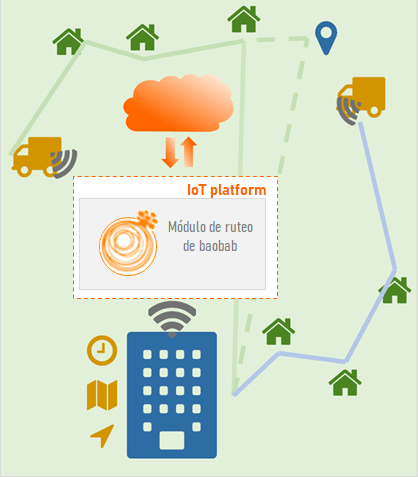
Tracking delivery vehicles using RTLS and appropriate sensors for each type of cargo, allows maximizing efficiencies on routes, especially in those cases in which vehicles must carry out deliveries and collections with little notice.
When delivery vehicles are en route, a routing module stores their location, their route plan (including addresses and time windows), the available load, and data of the personnel who attend the delivery: logged hours, preferences, etc.
If the system receives a new collection request, the module is able to propose the ideal vehicle to carry it out considering the impact on the existing planning, the load capacity of all vehicles and the work and rest times of the personnel, in such a way that a vehicle without space available is not assigned, nor is one whose driver has already finished his shift, or that affects the other deliveries.
Predictive maintenance and maintenance plan optimization
Monitoring the elements of a system (temperature sensors, vibration, pressure, etc.) together with the breakdown and failure logs allow the use of predictive analytics to anticipate failures before they occur, observing the patterns that follow the different magnitudes and comparing them with known patterns of previous failures, which make up the use case known as predictive maintenance.
Likewise, predictive analytics can help to adjust preventive maintenance deadlines, understood as the periodic reviews that must be carried out on the elements of a system even when no performance degradation has been detected. With a sufficiently large data set, these timelines can be adjusted without increasing the risk of failure.
These two use cases by themselves do not employ prescriptive analytics, which can be used to build the resulting maintenance plans, consisting of predictive, preventive and corrective maintenance tasks arranged in an optimal way, allocating each maintenance specialist the most appropriate tasks based on priorities, deadlines, material and necessary tools and, again, considering the training of the specialist , its schedule, conditions, etc.
The resulting maintenance plan will consist of the agile allocation of tasks to specialists in such a way that the priorities are taken care of with the smallest amount of time dedicated to supplying materials, components or tools, and downtime.