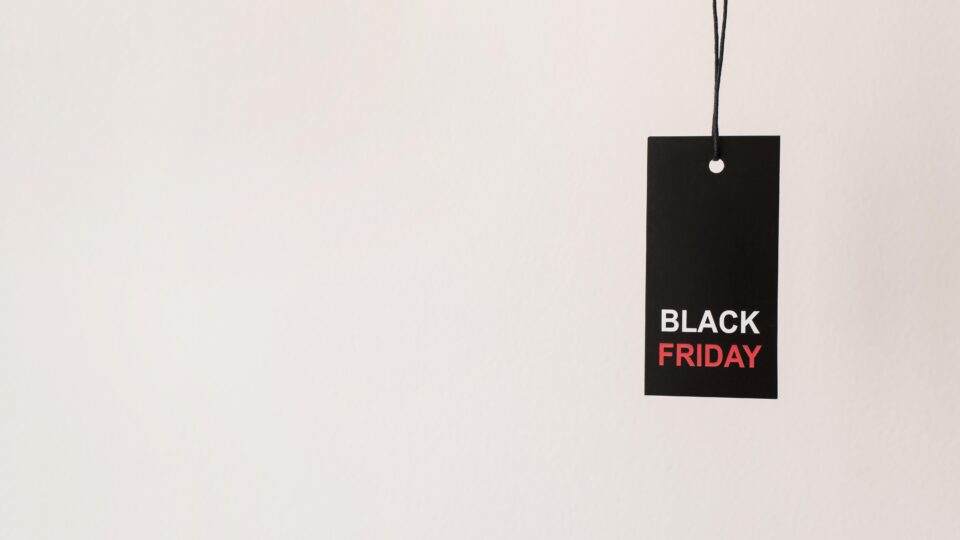
One of the most significant sales moments of the year is coming up. Everyone is preparing for this moment by loading warehouses, reinforcing teams in physical shops, reviewing e-commerce, data management, pricing, and picking or lead time to meet the expected demand. But are we doing it correctly?
So far, large and small retailers, physical or online, base their decisions and adjust to peak sales using various BI tools, usually mixed with the expertise and nose of the specialists they have at their disposal. Generally speaking, these profiles are estimated future demand based on past data. This process may be appropriate if no other option is available. But if there is one thing that day-to-day life is showing us, this is that the results of these estimates rarely coincide with what happens.
These estimates form the basis for sizing our resources or distribution channels. Although a proper estimation does not guarantee correct sizing, failing to make the right decision could hold back our growth in these sales peaks by 10% to 12%.
Another possible weakness of this part of decision-making is that different departments are often involved in this process, and many variables need to be managed to arrive at an optimal solution. Falling short on one decision will prevent us from realising the full potential of each sales peak; going too far will lead to cost overruns that will make it expensive to ride the wave of sales.
The best way to see the actual dimension of the decisions to be made in a peak sales situation is to list some key questions we should be asking ourselves:
- Which product should I display on my website, and in which order? Should I show the same products to all my customers or personalise my website views based on the data I have per customer?
- What is the best price I can put on each product at such a decisive moment as Black Friday? Which would be the optimal point of the discount quantity and elasticity of demand for each product?
- Do I have the capacity to take advantage of all the orders I receive through the web? Do I lack physical or digital resources?
- Do I have the proper inventory to handle all sales without overstocking my warehouses? Are my warehouse processes efficient? Do I have the right person in the right place?
- In the case of physical shops, what product should I stock in each shop and how should I manage replenishment? How many people do I need for my in-store process to be optimal?
- Am I working with the best possible logistics prices and routes? Are there unnecessary product movements or journeys? When should each product arrive in each shop?
- How many hours do I spend preparing and making decisions related to such events?
In our experience, these decisions are often made by different departments, based on historical data and the experience of their profiles. But can you imagine a dynamic, centralised tool, addressing all the variables simultaneously, that could answer all these questions and also provide you with alternative decisions that had not occurred to you?
There is no need to imagine anything because these solutions already exist and are successfully applied in sectors such as logistics, industry, energy production and distribution.
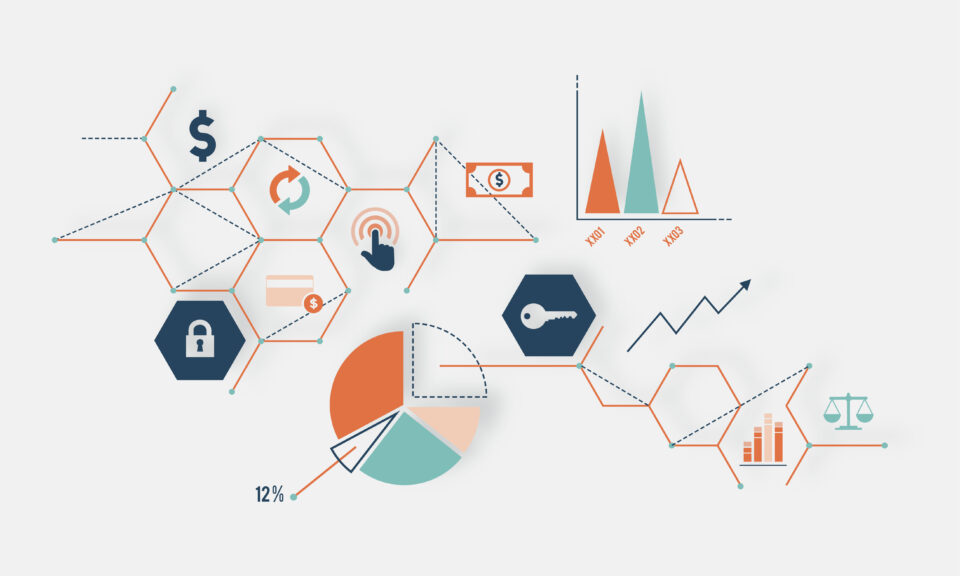
It is about Artificial Intelligence applied to each business, based on algorithms that current BI tools may initially nourish, but to which we could add market, competition and economic cycle studies, to build the “perfect machine” that will give us future solutions, providing the systems with very high levels of effectiveness and efficiency.
Although there are many examples, we will talk about a well-known retailer of American origin, a reference in international fashion with more than 100 years of history. To give some context, we summarise its business units into three: physical shops, wholesale (marketplaces and distributors) and online shops. The integration of their business units and Omni-channeling are objectives that they have not been able to achieve.
Currently, retail shops, with more than 1,000 shops worldwide, are the largest and most cost-intensive leg of the business. They have a BI system that has been collecting data on the performance of all their products, materials and campaigns for more than 15 years. In addition, they store a lot of customer information through their online shops, and loyalty programmes and have offices in all the countries in which they operate, reporting to two global headquarters, one in the US and one in Central Europe.
From an operational point of view, each country headquarters has numerous departments in a matrix structure, so that the sales outlets receive their directives directly from the regional managers, but also the regional manager’s controller the local product managers, who in turn receive guidelines from their HQ counterparts who also control production.
From the point of view of in-store resources, the management of each shop is responsible for assessing the staffing needs at any given time, sizing their internal warehouse structures and distributing their product display by genre, type, etc. The company does not have its warehouses in the countries and delegates the warehousing and picking of its products to its logistics providers in each country, based on orders from local controllers.
100% of the company’s departments work on BI information, estimating their needs from the previous year’s data, and a great deal of the decision burden is placed on the expertise of the controller, local management and shop management to delegate their general stock management to the shipping provider.
Without having mentioned production, and focusing on one country only, we are leaving the lead time resource management (and with it the associated costs), the pricing, the inventories, the sizing of warehouses or the structure of the shops in the hands of highly skilled people who, first of all, rely “only” on their interpretation of the data, and secondly, who are not looking at the same picture and are not operating in tandem. The result is that getting all the decisions involved in this process of sizing a sales peak right becomes an act of faith, and potential efficiency leaks multiply at every link in the chain.
In short, there is room for much improvement in forecasting methods and a shift to deciding on a dashboard of options with corresponding impacts.
At baobab soluciones, we have been working for more than 10 years on creating this type of AI-based tool to improve the company’s efficiency.