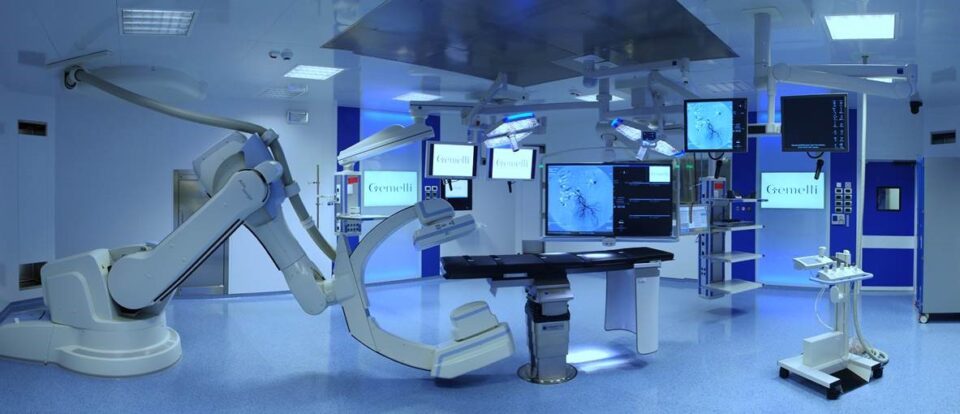
The average times on the waiting list for surgical interventions by the National Health System (SNS) in Spain began to increase around the year 2012 and, after slight fluctuations, reached 121 days as of December 31, 2019, according to official data. In more detail, for certain types of interventions the highest mean values reach 333, 163 and 137 days in plastic surgery, neurosurgery and traumatology, respectively.
If the same data is analyzed by Autonomous Community, we can observe differences of up to 110 days in average waiting time between different communities (La Rioja / Madrid versus Andalusia).
The planning of surgical interventions is currently carried out through a similar process in virtually all hospitals. This process consists in an initial arrangement of operating rooms for each service that, after receiving information on the availability of operating rooms, plans their interventions individually and in isolation.
Once each service is planned, the schedules are combined and revised by one central medical committee that detects the incompatibilities that might appear. These incompatibilities can be due to multiple reasons: lack of staff, overlapping material needs, etc.
It is important to highlight that during this planning stage, other factors related to productivity such as coordination with cleaning staff or caretakers are not addressed, which may cause delays or downtime in the operating rooms. In addition, the availability of resources from other related hospital units, like the sterilization or radiology units, is not considered either.
The combination of the aforementioned performance data and the gaps observed in the current process show that the programming of operating rooms considering the availability of resources can benefit from Prescriptive Analytics in order to reduce waiting times.
If we had to analyse all the different schedules (and allocation of resources) that can be given in a hospital when planning interventions, we would get an enourmous amount of possibilities to evaluate. So high that finding the optimal schedule without the proper analytical tool becomes impossible.
As a result of the high number of possible schedules to be evaluated manually, and the fact that the initial proposal that the committee receives is made individually for each service, the programming of interventions in the operating rooms is not optimal in terms of productivity.
How can Prescriptive Analytics help?
Prescriptive Analytics allows the development of a resource and surgery management system, that will offer optimal scheduling of interventions (in terms of waiting times), including their allocation to operating rooms and medical professionals, cleaning times for each operating room, orderlies and caretakers to transport patients and material resources from other units in the hospital.
Such a system would intelligently support the managers’ decision making and, eventually, would be in a position to carry out this task semi-autonomously under medical supervision.
As inputs, the system would use all relevant information about demand and resource availability. In general:
- The interventions to be carried out in each service, that is, trauma, cardiology, etc. and their characterisation (estimated time, material or need for personnel).
- The list of surgeons in all relevant services (traumatology, cardiology, urology, etc.) including their characterisation in terms of training, availability and preferences.
- The list of anesthesiologists, their characterisation and availability.
- Nursing staff, auxiliary and orderlies.
- The number of operating rooms associated with each service.
- Cleaning staff, their availability and the characterisation of their tasks.
- Any other needed resource.
All these data provide an immediate vision of the needs and resources available to the tool to carry out an efficient management of these services that includes the optimal planning of each operating room and the allocation of resources to each intervention. After feeding the tool with these data, it will systematically evaluate the solution space formed by all the possible combinations, intensifying the exploration in the most promising ones and diversifying the search to identify possible new attractive solutions so that, depending on the technique applied, it can obtain a plan that is optimal or very close to optimal.
Another advantage of the agility and versatility of Prescriptive Analytics is the flexibility it provides for replanning. Complications during an intervention are common, which cause delays and cancellations specially of operations planned for the end of the day. With the intelligence and planning speed of these Prescriptive Analytics techniques, the rescheduling of cancelled operations is much easier than it was with manual planning.
A possible technological solution could work as in the following diagram, which shows the input data that will have to feed the autonomous engine based on mathematical optimisation techniques and machine learning, the output data that will be displayed in the interface and the replanning loop.
Inputs and outputs of an operating room optimisation model.
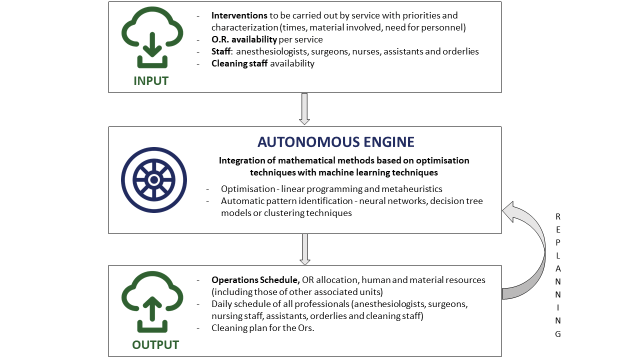
In connection with the autonomous engine, mathematical modeling techniques are applied based on the optimisation of the operation calendars. Specifically, linear programming, which is based on the definition of a set of variables subject to a series of restrictions and a linear objective function, the value of which must be maximized or minimized.
The systematic analysis of the space containing all possible solutions that satisfy the imposed restrictions favors a quick identification of the one that best achieves the predefined goal. In addition, in the case of complex systems such as the one studied here, it may be convenient to use metaheuristic techniques that, although they do not ensure obtaining the optimal solution, do provide good solutions using shorter computing times.
Second, we could combine these optimisation techniques with artificial intelligence techniques by means of machine learning. Specifically, pattern recognition techniques allow the detection of patterns in optimal schedules. The autonomous engine carries out a learning process through experience, which allows reducing computation times in future resolutions. We can propose algorithms such as neural networks, prediction trees or clustering techniques for the automatic pattern identification.
As for the output, the two mockups below represent one possible appearance of the web interface.
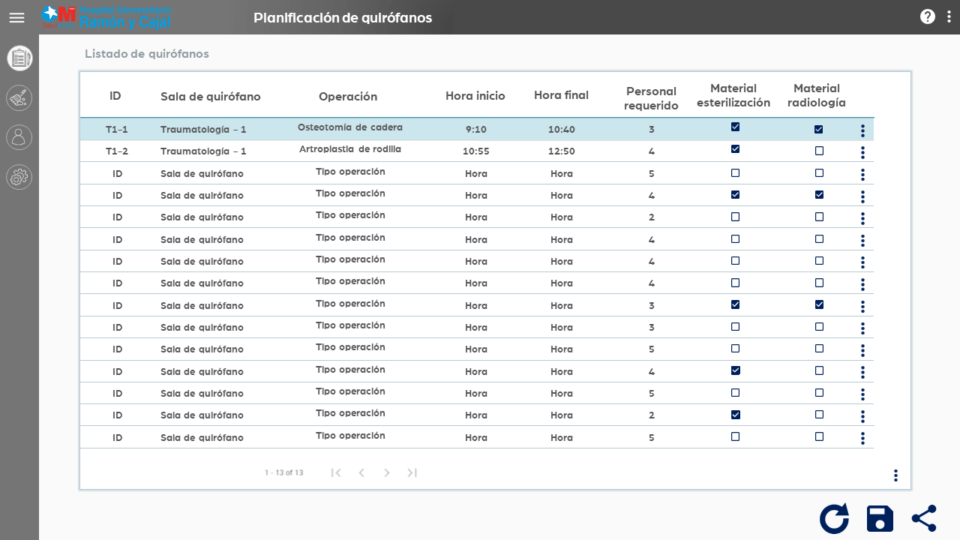
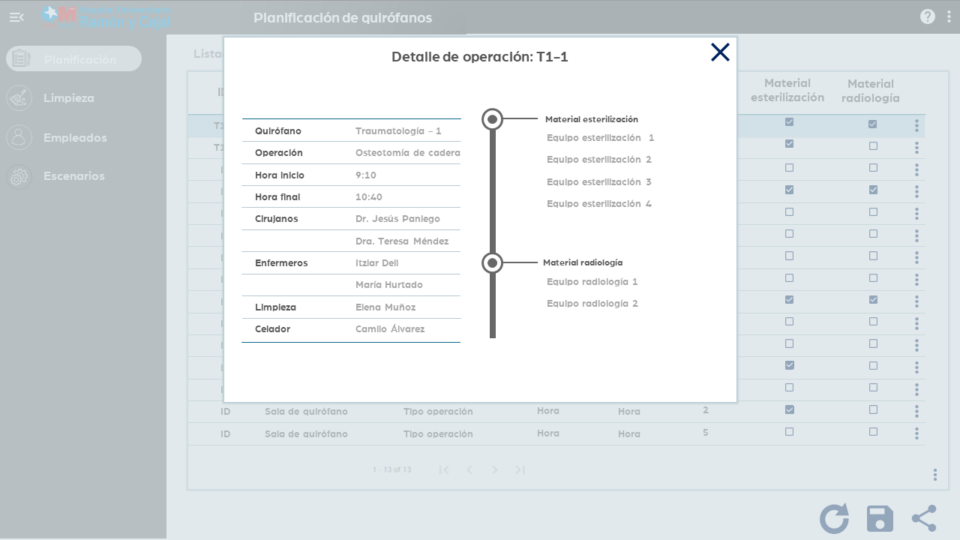
Conclusion – waiting lists can be reduced using Prescriptive Analytics
This concept involves the combination of Operations Research techniques and Machine Learning in the form of an intelligent tool, easily integrated into any system, which illustrates another use case of Advanced Analytics for Healthcare.