The energy transition towards renewable energies is inevitable nowadays, and in this process, advanced and predictive analytics play a vital role in its correct implementation in electricity networks.
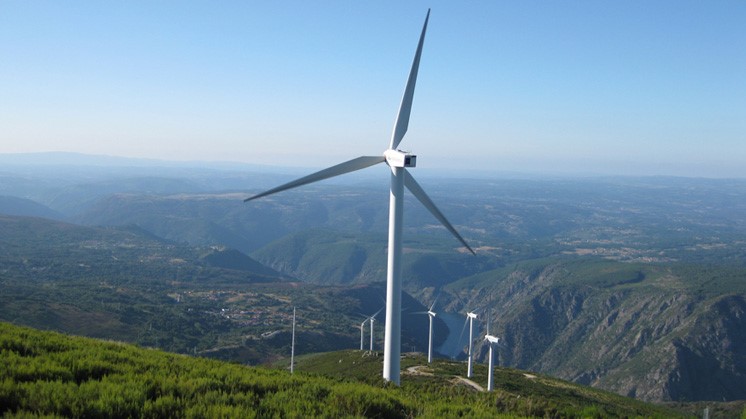
Continuing with the theme of the article on Artificial Intelligence in renewable energies, today we bring you one of the most important use cases of these techniques, focused on the correct implementation of these energies in electricity networks.
An electricity network is an extremely complex system that must balance demand and generation at all times. To do this, the network manager has models that allow him to estimate what the demand and production will be at any given time. These models will help to make the right decisions when it comes to managing the production that is fed into the network.
This is where the application of supervised machine learning and other advanced analysis techniques come in to develop predictive models to determine the future production of renewable energies. The most complex case, and the one on which this article will focus, will be the estimation of wind power production.
Predicting Wind Power Production Using Machine Learning Techniques
Creation of Predictive Models
As mentioned above, predicting wind production is a highly complex task. Wind generation depends mainly on the wind at the generation locations, so wind data are the main predictors of wind generation at a given time and will be the primary input to the models.
It is important to bear in mind that when we try to make future predictions, the data fed into the model are both past data already known, as well as future data resulting from forecasts. In the case presented, the meteorological models make these forecasts of different agencies.
As for the morphology of the data used, they could be defined as snapshots that define the module and component of the wind at each moment. These data are given on the different points of a grid that represents a geographical area as can be seen in the image below.
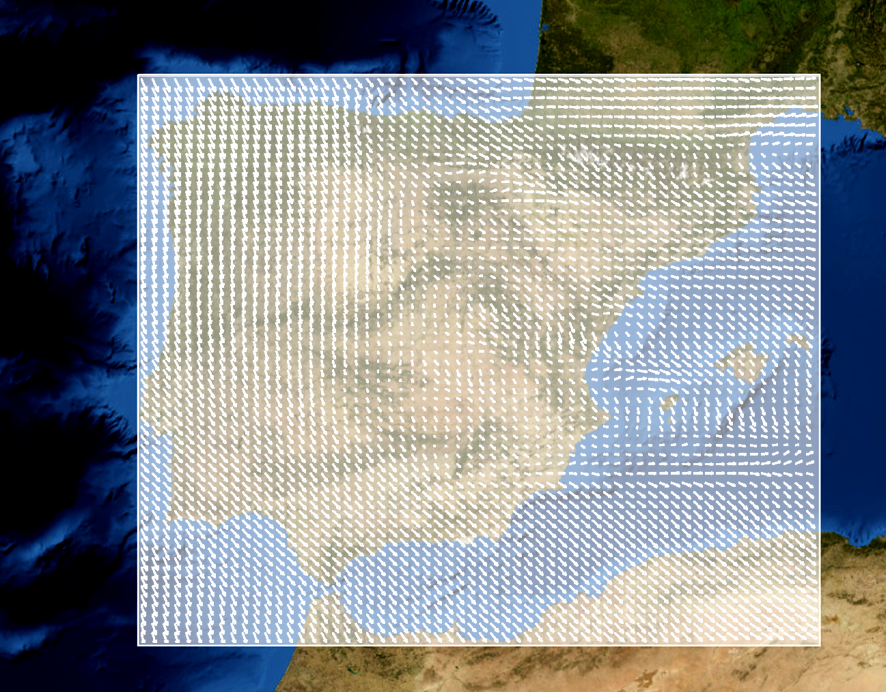
For the supervised learning process of these data, computer artificial visual techniques will be mainly applied using convolutional neural networks to analyse the snapshots and recurrent neural networks to analyse the temporal evolution of the winds.
Another relevant predictor, especially when creating short-term forecasting models, will be past production. These past outputs can be used in different ways, from being used as a simple starting point to calculate future outputs to time series analysis using statistical techniques such as ARIMA or SARIMA and even LSTM recurrent neural networks.
Main Sources of Error in Models
Despite the good performance of these models, the transition to renewable energies, in turn, generates new problems that affect the accuracy of the prediction models.
For example, with the increase in the amount of renewable power installed in an electricity network, there is also an increase in the frequency of specific moments in which part of renewable production has to be reduced or stopped. These point phenomena are due to various causes such as overproduction, overloading of certain high-voltage lines or maintenance of wind generators.
This leads to a considerable point loss in the predictive ability of the models as the prediction will be higher than the actual output. This is currently one of the main sources of error in prediction models.
Another important source of uncertainty is the error in the data used by the models. Mainly wind data are derived from the models of the meteorological agencies, which do not report the error that these data usually have.
Why is it Important to Improve Electricity Production Forecasts?
- Good forecasts are essential for the good performance of network managers and to organise production properly.
- If the installed renewable power is to continue to increase, it is essential to improve forecasts. Model error is measured as a percentage, and as installed capacity increases, the absolute discrepancy between real and predicted capacity will also increase.
- Providing wind farm managers with better data will also help them to improve their organisational capacity. They will therefore be able to carry out better planning by avoiding the costs of forcing an unexpected reduction in production and by adjusting maintenance outages to the most convenient times.
- Ultimately, network users will also benefit. Firstly, they will have access to a higher quality supply, i.e. with fewer fluctuations. In addition, electricity prices will be more in line with actual production because the accuracy of the power auctioned daily will be improved.
How Can Prescriptive Analytics Help Improve these Predictive Models?
Creating Reduced Production Period Estimation Models
Advanced analytics could have a major impact on improving the predictability of wind power production by helping to predict the point in time when production is reduced.
With the available production data and forecasting models accompanied by real-time and historical information on the state of the electricity grid, production could be analysed to determine when this reduction in production occurs and thus determine the most influential factors.
Once the models that determine these exceptional moments have been obtained, they could be implemented in wind prediction models to correct for the influence of these exceptional situations.
Improve Uncertainty of Input Data
While it is true that the use of neural networks tends to smooth the variability of the input data through the use of learning algorithms such as gradient descent and its derivatives, this does not detract from the fact that by working with better data the models can be substantially improved.
Currently, one of the major handicaps in wind analysis and management is the lack of meteorological data. Despite the efforts made to standardise and encourage data collection to facilitate and improve wind management, data are still scarce.
Thus, with the right knowledge and tools, joint strategies for meteorological data collection at generation sites could be developed. This data, using data science and advanced analytics, could be used not only to improve forecasting models but also to enhance decision-making and evaluate potential sites for new wind farms, among other uses.